AI for Industrial Use Healthcare Products
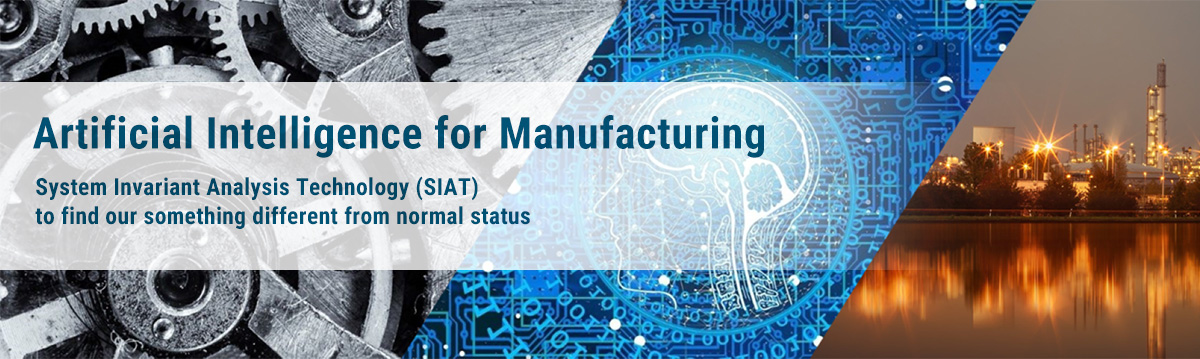
Application
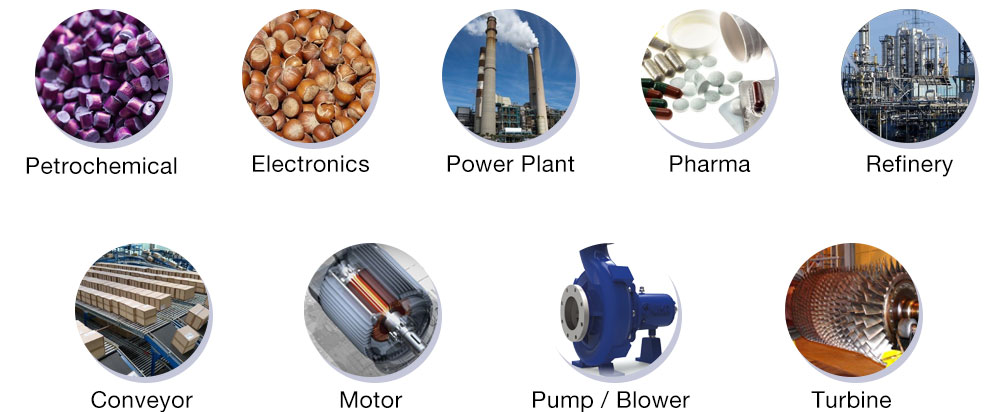
Anomaly / Abnormal Detection and Predictive Maintenance
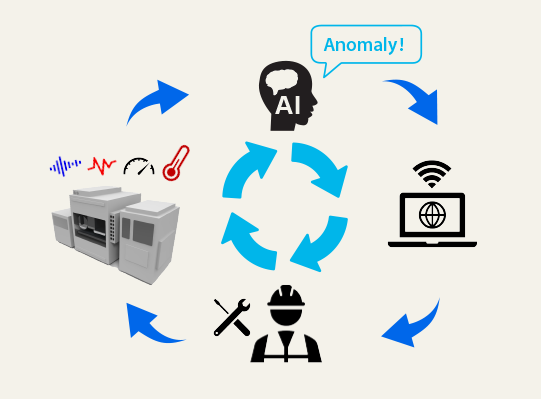
-
Detect the signs and symptom of machine failure before critical damage
-
Eliminate excessive regular machine maintenance rounds
-
Minimize maintenance cost
-
24-hour machine monitoring
-
Beforehand preparation and smooth spare parts replacement.
Reduce or eliminate machine down time
-
Remote condition monitoring
-
Many parameters can be measured and monitored at one place
Product Quality Control
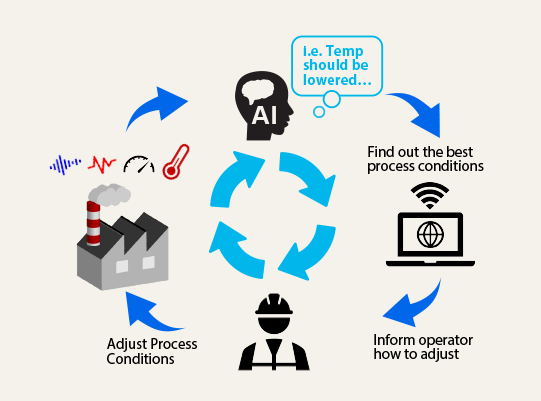
-
Detect process condition variation from normal operation parameters
-
Maintain consistent and best quality of final product
-
Reduce or eliminate NG product
-
Increase productivity and reduce man hours
-
Remove man dependent process factors
Visual Inspection
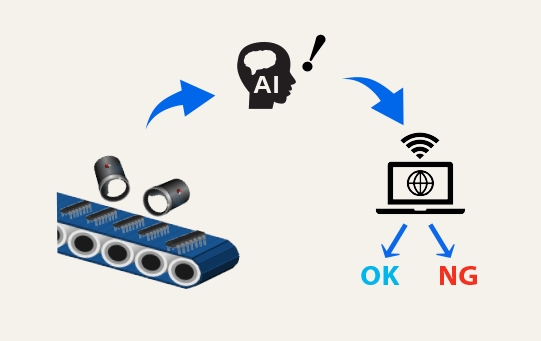
-
AI to distinguish OK or NG way better than conventional threshold judgement
-
Decrease miss judgement of OK or NG resulting in higher yield
-
Reduce workers and cost for inspection
-
Increase through put
-
Reduce human dependent error. Eliminate human dependent quality check level
How It Works?
All copyright belongs to NEC Corporation
Extract invariant relationships from time-series data consisting of multiple sensors and create a monitoring model. Invariant analysis technology that detects abnormalities from the current sensor time series using that model.
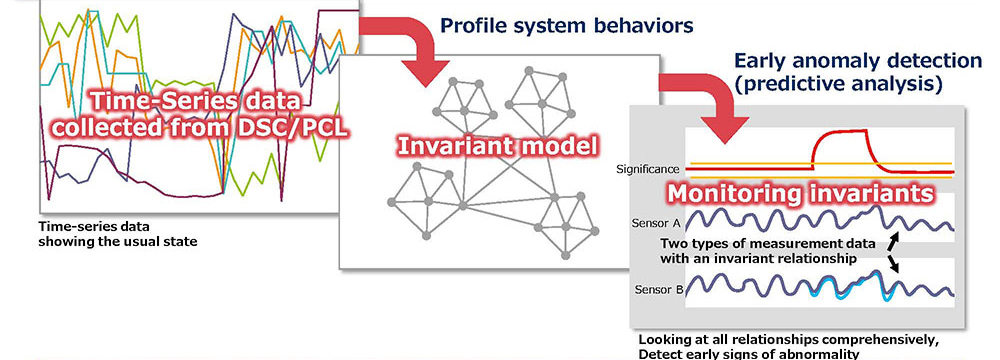
- 1. Each relationship captures local characteristics of the target component.
- 2. By finding a large amount of relationships, the characteristics of the entire system can be found from a different viewpoint than before.
- 3. It is possible to understand the operation status of the system by monitoring the change of each relationship.
Case Studies
Case 1 – Space Domain Application
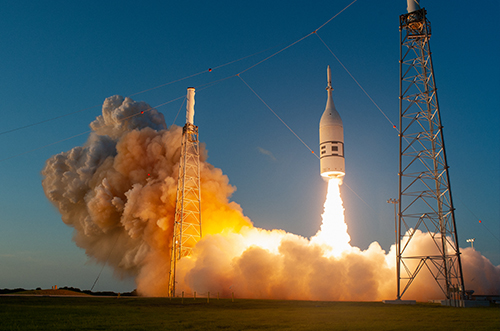
-
Early production testing and operational scenarios studies (before its actual mission in space).
-
Make it possible to do proactive anomaly detection during design, development, production and test stage. And created system-level Digital Twin.
-
Built a model of vehicle’s normal operation from about 150,000 sensors to establish over 22 billion logical relationship for analysis.
Testimonial by Rockheed Martin. All copyright belongs to NEC Corporation
Case 2 – Automotive Components
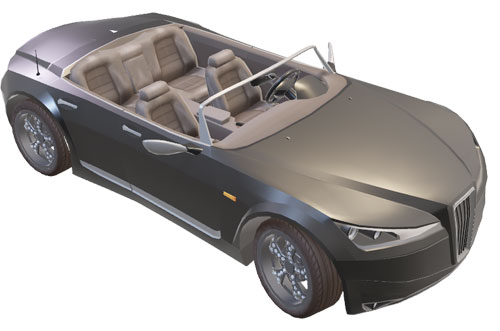
Before | After | |
---|---|---|
Impossible to monitor Press Components quality in real-time | ![]() |
Achieved real-time monitoring. |
Excessive redundant machines in case of breakdown. | ![]() |
Achieved anomaly detection and reduced number of machines. |
Could not find root cause since took time and power only to find out the location where causes defects. | ![]() |
Analysis of defective parts by AI using collective data and explored into root cause analysis by operators. |
AI solution was deployed to automotive press parts production process. Further to abovementioned improvements,
✓ Customer succeed to launch AI used system within 5 days from its incorporation.
✓ Optimized machine repair and spare parts replacement cycle preventing unscheduled repair work.
Case 3 – Injection Molding Machine
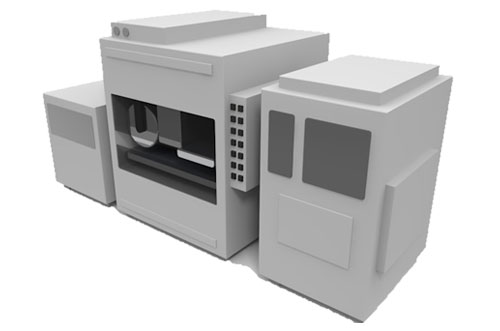
Before | After | |
---|---|---|
Defection was found considerably later than when the failure happened. | ![]() |
Achieved real time detection of defection by monitoring unusual status. |
Defective products were disposed though those appearance was good. | ![]() |
Realizing early detection before by the defects to happen, considerably decreased defective products. |
Noticed machine failure after finding NG product. | ![]() |
Able to condition and do maintenance before producing NG product. |
This customer used to notice the defective parts after the anomaly of production machine and kept producing defective products though the machine is already unusual. After introducing AI system, customer could find anomaly of injection machine way earlier than before. This resulted in higher productivity and lower disposition of NG products.
Case 4 – Refinery
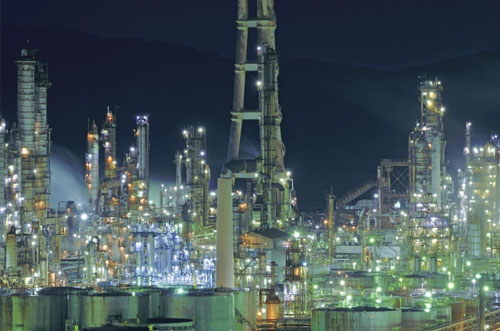
Before | After | |
---|---|---|
To realize early failure detection system to be an advanced technology utilizing refinery. | ![]() |
Found important relationship among over 500 sensors (temperature, pressure, flow, valve position and water-level in boilers). |
Defective products were disposed though those appearance was good. | ![]() |
Spent lots of time and workforce to analyze root cause but couldn’t set up effective system maintenance plan. |
This AI solution allows the customer to find failure indication 1 week earlier than previous system based on thresholds and trend analysis and minimize the impact.
Track Records
Sector | Customer | System Contents |
---|---|---|
Power Plant | JERA Co., Ltd. | Monitoring of signs of failure for thermal power plant, monitoring of efficiency degradation, and visualization of operational status, and operation Integration (4 power plants) 5 units of coal-fired steam power and 20 units of combined gas turbines |
Power Plant | Medco Power Indonesia | Monitoring of signs of failure at thermal power generation facilities (GTCC) and making maintenance works more efficient |
Energy | JXTG Energy (Mizushima Refinery) |
Reduction of workload and optimization of maintenance plan by detection of abnormal signs of utilities and early maintenance |
Petrochemical | Sumitomo Bakelite Co., Ltd | Detection of anomaly signs and automatic control of process conditions enabling stabilization of product quality |
Steel | Nippon Steel Corporation | System failure anomaly monitoring and Real-time quality monitoring in manufacturing process |
Space Exploration | Lockheed Martin | Reduction of development cost and improvement of safety for spacecraft development. Improvement of safety by monitoring sophistication. |
Railway | JR East | Stabilization of operation by detecting signs of failure of railway vehicles and improving the efficiency of maintenance |
Other | JAXA Aerospace Exploration Agency (joint research) | Engine Abnormality Monitoring of Reusable Rocket and Its Use in Aircraft Inspection System |
Other | Tohoku Univ. (Joint research) | Inspection efficiency improvement by utilization for non-destructive inspection |
Other | Tohoku Univ. (Joint research) |
Stabilization of quality during material production |
